

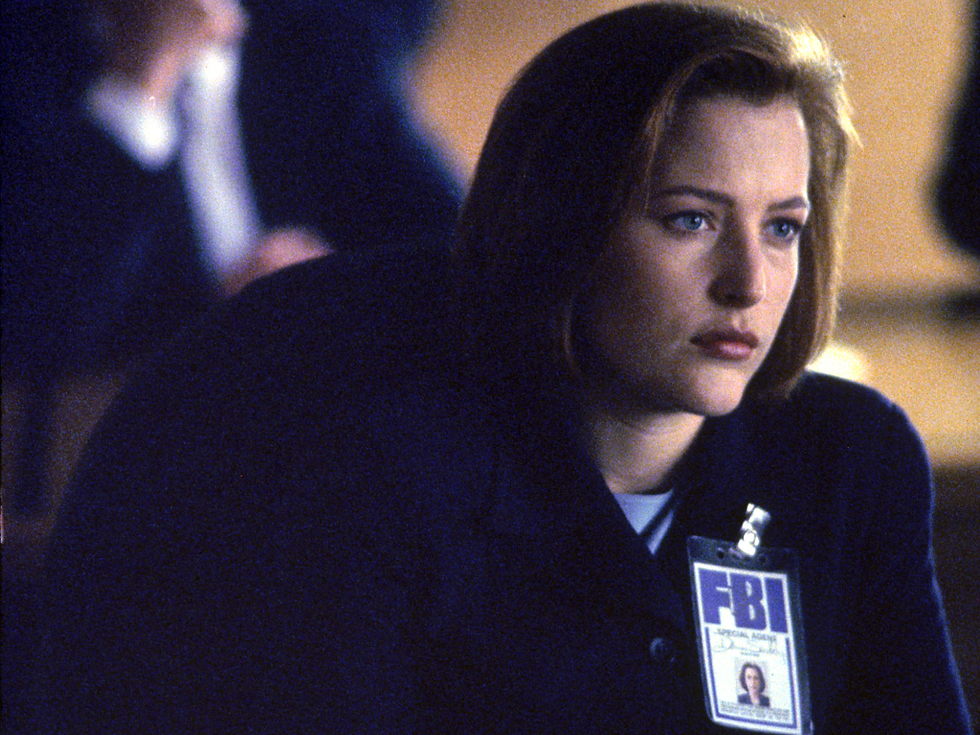

BACKGROUND & MOTIVATION
When I was a young boy, my sister mysteriously disappeared. This has haunted me for my entire life and I have never stopped searching for her. After graduating from the University of Oxford, I joined the FBI and worked in the Behavioral Science Unit and then the Violent Crimes Unit. It was during this second assignment that I discovered (and became obsessed with) the X-Files, a section of the FBI devoted to investigating cases related to the paranormal. For a while I investigated these mysterious cases alone, until I was assigned a partner from the FBI Academy's Forensic Science Research and Training Center: Dana Scully. I believe in extraterrestrial unidentified flying objects (UFOs) and a government conspiracy to deny the truth of their existence; Scully is the voice of scientific reason to my theories. I need her to believe that UFOs are real so that she can help me find and bring back my sister.


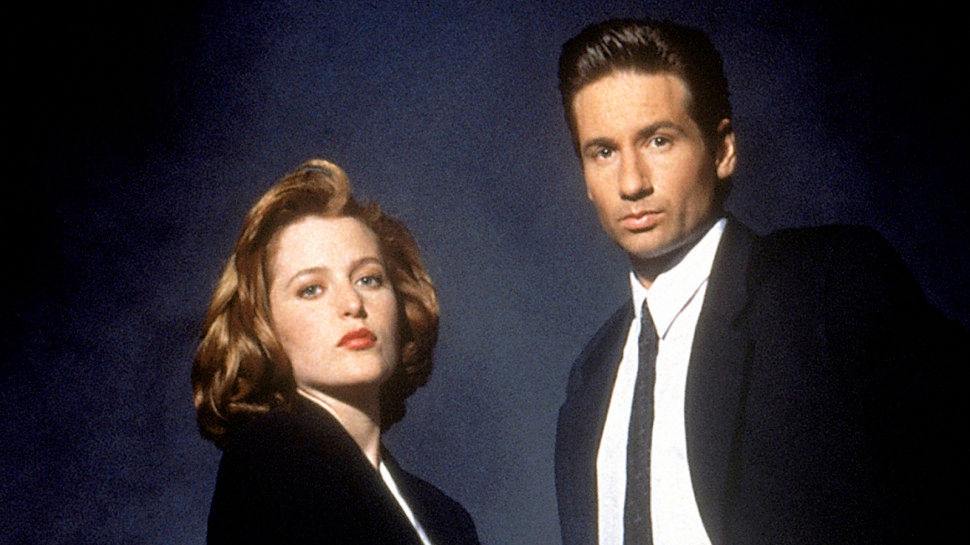
OBJECTIVE
I need to know whether or not UFOs are real. In order to reach a conclusion on this matter, I will conduct exploratory analyses and create visualizations of existing data on UFO sightings from the National UFO Reporting Center and then use these data to construct models to predict the shape of future sightings. By conducting this work, I will hopefully be able to lend credence to my existing belief in UFOs and convince my partner, Dana Scully, as well as the rest of the world, that UFOs are not only real but also predictable. Scientifically, establishment of such a pattern would not only further acceptance of the reality of UFOs but serve as the foundation for future investigation into and better understanding of extraterrestrial life. On a personal note, conducting these analyses and systematically convincing Scully of the reality of UFOs is the best chance I have of getting my sister back.
THE DATA
56,306
ufo
sightings
Earliest sighting:
December 28th, 1925
116 HOURS = LONGEST
<1 MIN = SHORTEST
FIRST POST ON 03/07/1998
Click on a plot for a closer look.
![]() | ![]() | ![]() |
---|---|---|
![]() | ![]() | ![]() |
![]() |
There appears to be a tremendous increase in the number of sightings per year in 1998, which is to be expected, since that's when the National UFO Reporting Center started posting reports online. Since 1998, the number of reportings per year seems to have increased steadily, with large jumps around 2011 and 2014. Across time, it appears that UFO sightings were most frequent in the summer and fall. When I examined UFO sightings by month, I found a huge increase starting in May and peaking in July (corresponding to the summer seasonal trend). The lowest number of UFO sightings occurred in February, also consistent with the winter seasonal trend. I decided to also look at the day of the month. I wasn't expecting to find anything, however, to my surprise there was a large increase in sightings that occurred on the 1st and 15th of every month. There doesn't appear to be any association between day of the week and number of sightings reported, although there are slightly more sightings on the weekend, specifically on Saturday. The most popular time to see a UFO was 9 PM. The majority of the UFO sightings occurred between 7 PM and 12 AM.
![]() | ![]() |
---|
TOP FIVE CITIES FOR TOTAL NUMBER OF SIGHTINGS
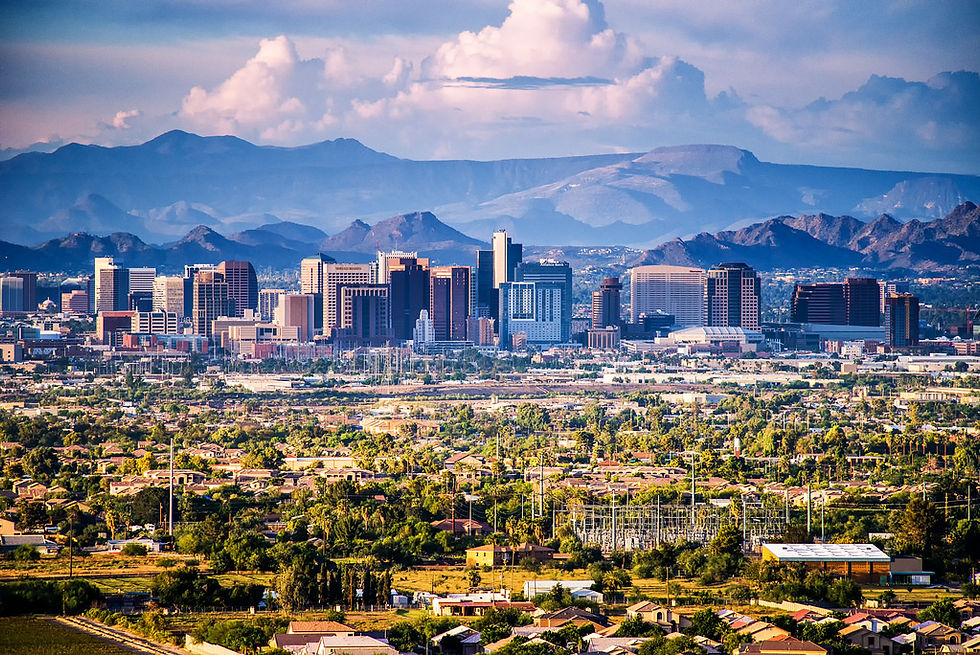



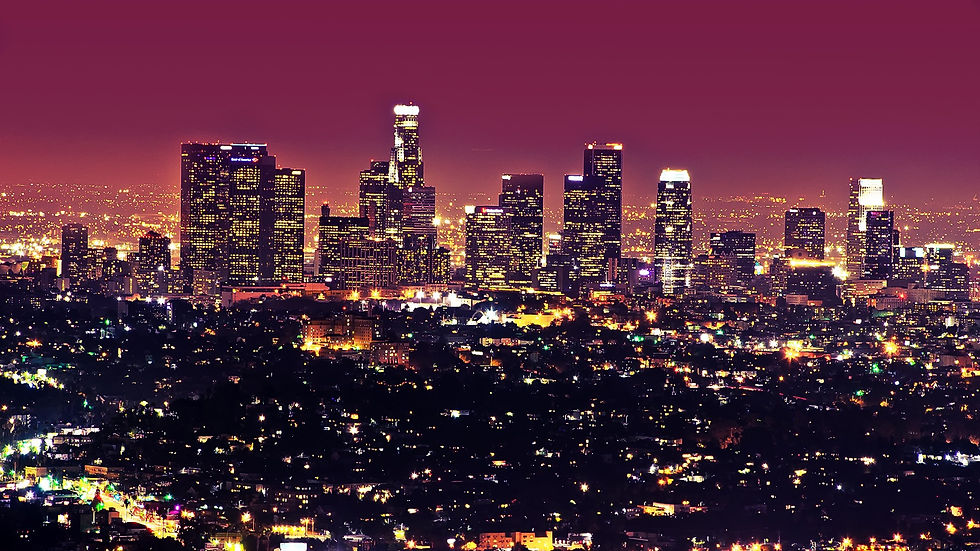
TOP FIVE CITIES FOR TOTAL NUMBER OF SIGHTINGS PER CAPITA
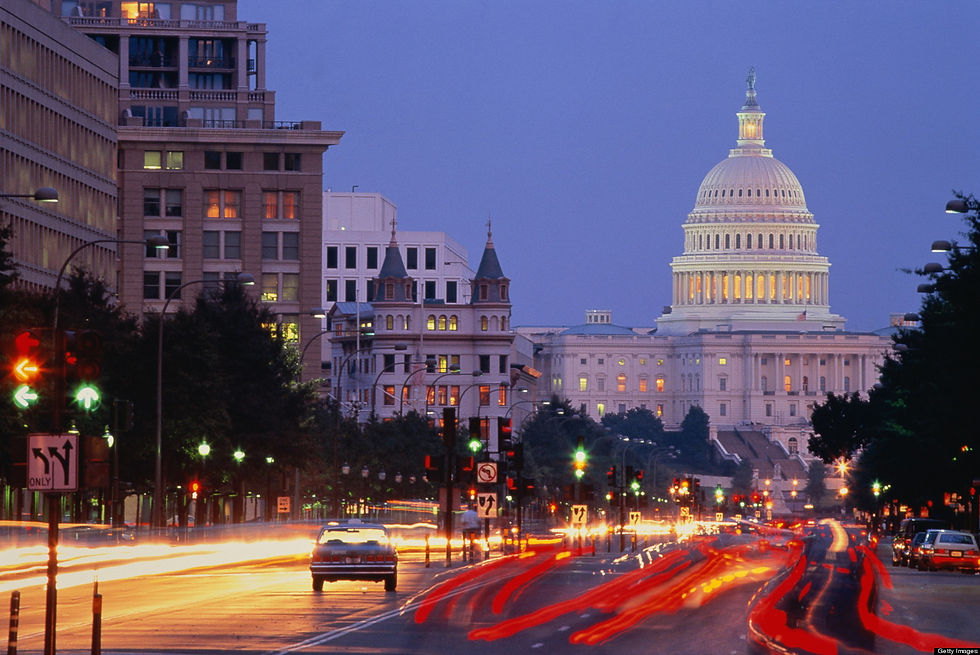


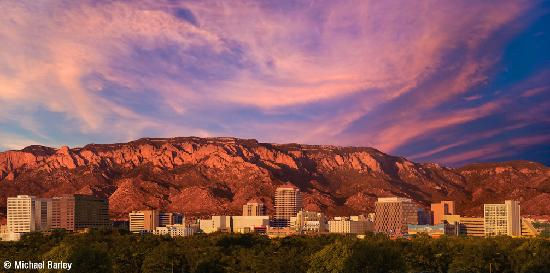
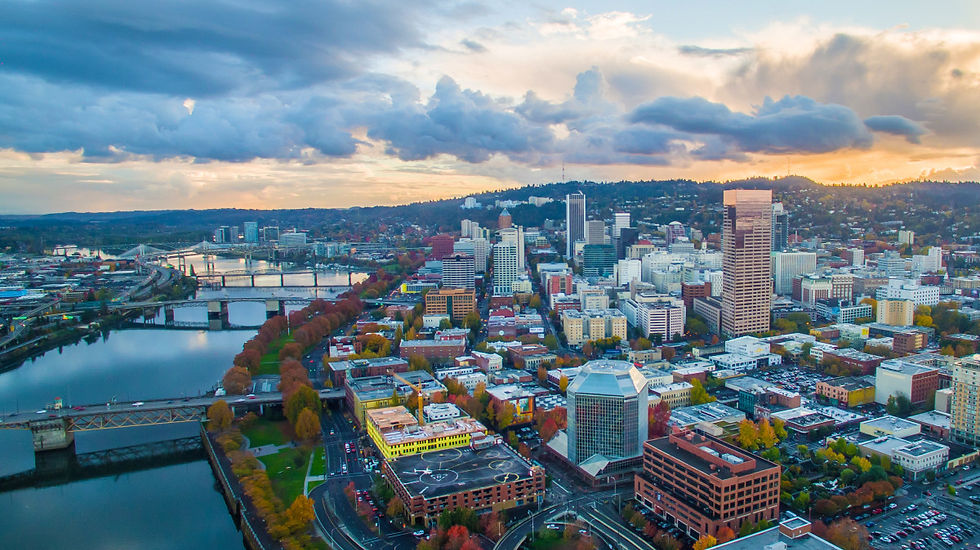
![]() | ![]() |
---|
Since I knew that states with larger populations were more likely to report more sightings than states with smaller populations, I decided to look at both the absolute number of sightings reported and the total number of sightings reported per capita. I used 2010 population data because the most sightings were reported in the 2000-2010s and the state populations were relatively stable across that time period. I found that California and Florida reported the highest number of total UFO sightings, while New England and the Pacific Northwest reported the highest number of per capita UFO sightings. This was also reflected in the top five cities for both total number and total number per capita of reported UFO sightings. These state trends were also consistent over time.
![]() | ![]() | ![]() |
---|
It appears that round, triangle, and oblong were the most common shapes. While most of the country reported round UFO sightings the most, Maine and Rhode Island both reported fireball, North Dakota reported oblong, and Iowa reported triangle UFO sightings the most. The most common shape sightings were fairly consistent over time, however, the fireball shape experienced a substantial increase in number of sightings between 2010 and 2015.
![]() | ![]() |
---|
It appears that most sightings were approximately 1 minute (or less) in duration. The majority of the sightings were under 10 minutes. There doesn't appear to be any sort of relationship between shape and duration, although it seems like fireball has more 1 minute (or less) sightings and changing has more >10 minutes in duration sightings.
​
The barely existent association between shape and duration put a damper on my spirits. I decided to turn to Scully for advice. Always the skeptic, she suggested that I look at weather data and see if any of the sightings could be attributed to local weather patterns.
![]() | ![]() | ![]() |
---|---|---|
![]() | ![]() | ![]() |
![]() | ![]() | ![]() |
![]() |
The most sightings occurred during a clear night, followed by a clear day, and then a partly-cloudy night. This was fairly consistent after stratifying by shape. Round was the predominant shape for all weather patterns except for fog and snow (both oblong), but oblong was the predominant shape for these two weather patterns with only 4 and 1 reported sightings, respectively. It appears that diamond and triangle sightings were most concentrated between very light and light precipitation intensity while oblong and rectangle made up a majority of the sightings that occurred during light to moderate precipitation. Almost no sightings occurred during heavy precipitation. The distribution of shapes by precipitation probability showed that a majority of the oblong sightings occurred when the precipitation was ~50% and most of the diamond sightings occurred when the precipitation was ~15%. Supporting the other precipitation-related data, diamond sightings were greatest when the cloud cover was ~35%. Unfortunately, there were no real differences in the distributions by shape for actual temperature, relative humidity, wind speed, and pressure.
​
It should be noted, however, that these findings should be interpreted with caution, as I was limited in how much weather data I could actually get my hands on and only had a total of 1,869 UFO reports to work with for these analyses.
![]() | ![]() | ![]() |
---|
Looking at the distribution of percent of sightings within a year over time (by month & year), it appears that sighting percent may be influenced by the release of space-related movies and the occurrence of major financial crises and threats or attacks. For instance, there seems to be a large spike in the percentage of sightings within 1982 at the time of the release of the movie ET. Similarly, there is spike in percentage of sightings for 1981 near the date of the onset of the 1980s recession. The incidence of major threats and attacks, however, matches dates of increased percentage of yearly UFO sighting reports most closely. From the plot of percent of sightings relative to major threat dates, you can see that for almost every major threat or attack there is a corresponding point of disproportionately high percentage of sightings for that year.
![]() | ![]() |
---|
"Light", "object", and "sky" were the most commonly used words to describe UFO sightings. Interestingly, there was an average of approximately one "trust"-related word used in every two summaries and most summaries were, on the whole, positive descriptions.
MOST POSITIVE REPORTS

a rising or nearing bright yellow glowing rectangle stopped to create a fireball shape dropped glitter like substance and disappeared

fireball green with white center with a tail of white sparkling stuff it went horozontally across sky winked out
MOST NEGATIVE REPORTS

i was outside when these big fireballs came neer my house i took out my shot gun and shot at them they came back and shot theyr guns at...

a small gray saucer on golf course they where pulling at me they wanted me to go with them i got very sick from attack n...
THE ANALYSES
What more could I learn from these descriptions? Perhaps, I could use the words in the text summaries of the UFO reports to predict the shape of the UFO using a naive Bayes model. A naive Bayes model attempts to predict what category (or class) a variable of interest belongs in, given a sample of data, using Bayes' theorem (although, technically, it provides a probability distribution over a set of categories, not just the probability of the most likely category). My "variable of interest" is UFO shape. It is naive because it makes the very strong assumption that the other variables that you think might help classify the variable of interest (the "features") are independent of one another. My "features" in this analysis are the individual words in the text summary field of the UFO reports -- which we can clearly see are not independent of one another.
26%
Unfortunately, there doesn’t seem to be any underlying insights into the shapes of UFOs that are contained in the summary descriptions, as I can only predict the shape of the UFO sighting with approximately 26% accuracy. While the naive Bayes model does manage to do a better job than simply guessing one of the 12 shapes at random, it does quite poorly. I could do better just by betting than any UFO is round!
​
Perhaps, I can use my findings so far and build a multinomial regression model to predict shape. A multinomial regression model is like a logistic regression model, except it is applied to a discrete outcome variable (e.g., shape) with more than two possible outcomes. This model will predict the probabilities of the different possible shapes, given a set of independent variables (like the features for the navie Bayes analysis). The independent variables that I chose to model for this analysis included the year, season, day of the week, hour of the day, and duration of the sighting; the number of days between the reported sighting and when the sighting was posted on the National UFO Reporting Center website; whether the sighting occurred in a state whose most frequently sighted UFO shape was not round; and whether or not the sighting occurred close (in time) to a UFO or alien-related movie release date, a financial crisis, and/or the occurrence of a major threat or attack.
36%
While the accuracy has improved over the text predication using naive Bayes, the overall accuracy still isn’t very high. Most notably, it appears that the model overwhelmingly predicts round as the UFO shape. As such, the accuracy level of the model is likely attributable to relatively high proportion of round shaped sightings.
In an effort to find a more accurate model, I think I’ll try k-nearest neighbors. K-nearest neighbors (knn) is another classification model. This model takes new data and tries to classify it based on data that it already has by calculating some kind of similarity measure (e.g., Euclidean distance). The value of this similarity measure is then used by the model to predict how the new data should be classified. In my knn model, I predicted shape classification using the following features: the year, month, day of the week, day of the month, hour of the day, and duration of the sighting; the number of days between the reported sighting and when the sighting was posted on the National UFO Reporting Center website; and what region of the U.S. the sighting occurred in.
35%
While the knn model does not quite as overwhelmingly favor the round shape category, this model is not much of an improvement over the multinomial logistic regression model.
I’ll give it one last go with a random forest analysis. Random forests are a type of decision tree. Decision tree models where the target variable of interest (e.g., shape) can take on a finate set of (discrete) values are called classification trees. The "leaves" of these classification trees represent the values of the target variable (e.g., the different shape categories) and the "branches" represent the combination of features that led to those value labels (e.g., varying combinations of hour, year, and duration of sighting...etc.). By design, decision trees "overfit" or "overtrain" their (training) data because the resulting classification tree is a direct result of the observed data in the training set. Random forest models correct for this problem of decision trees overfitting their data. I will run my random forest model with the same set of features as I used for my knn analysis.
![]() |
---|
35%
The random forest analysis is very similar to the multinomial logistic regression and knn analyses. Interestingly, it appears that difference between the observed and posted date of the sighting has the greatest impact on the random forest analysis.
THE TAKEAWAYS
Now that I have completed my analyses, I need to summarize them in order to formally make my case to my partner, Scully. From my exploratory analyses of these data, I learned that:
​
-
after removing invalid shapes & cleaning the data, there are over 56,000 reported UFO sightings that occurred from 1925 to 2016
-
the number of reported sightings has steadily increased since the database first opened in March, 1998
-
sightings most commonly occur in the summer and fall, specifically between June-November
-
within each month, sightings most commonly occur on the 1st and 15th days of the month
-
sightings are most commonly reported on Fridays, Saturdays, and Sundays and between the hours of 5PM-1AM
-
California has the highest absolute number of reported sightings
-
after adjusting for 2010 population size, states in New England and the Pacific Northwest had the highest per capita reported sighting frequency
-
the most commonly reported UFO shape was round, followed by triangle, oblong, and fireball
-
the duration of sighting varied by reported shape
-
most sightings were reported on clear nights, which is confirmed by the low probability of cloud cover or precipitation
-
actual temperature, relative humidity, wind speed, and air pressure varied only minimally by reported UFO shape
-
the most commonly used word in the UFO report summaries was light, followed by object, sky, and shape
-
text based summaries displayed a range of negative to positive sentiment scores
-
comparing the frequency of reported sightings by date to dates of major events, it appears as though increases in report frequency may correspond with the release of space-related movies, financial crises, and/or major threats and attacks
From these observations, I believed that it might have been possible to predict UFO shape and used a variety of methods to try to do so. While I found many statistically significant predictors (or features) of UFO shape, I am only able to predict UFO shape slightly better than chance. At best, using multinomial logistic regression, I was able to predict UFO shape with only 36% accuracy.
​
In spite of my inability to predict UFO shape with high accuracy, I still believe in extraterrestrial unidentified flying objects and in a government conspiracy to cover up their existence. The trends in sighting times, locations, and conditions suggest that the reported sightings cannot be occurring simply at random and that there must be some intelligent life forms out there behind these UFOs and that they might know what happened to my sister.
SCREENCAST
ABOUT US

AMANDA ANDERSON
Kth-nearest UFO Observer
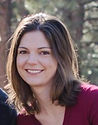
REBECCA BUTLER
(Least) Naive Bayesian

SAM MOLSBERRY
Regressing toward the Extraterrestrial